Though artificial intelligence (AI) and machine learning (ML) have existed for a while, their value and use have recently risen dramatically. Many people believe the two terms refer to the same thing because they are frequently used interchangeably. Yet, in reality, they are separate concepts with unique characteristics, purposes, and applications. To better understand AI and machine learning, we will examine their differences in this post and offer a comprehensive comparison.
Machine learning vs Artificial Intelligence
While they are frequently used together, machine learning and artificial intelligence are not the same. A Broader concept about Machine learning is a subset of artificial intelligence (AI), a broader concept AI is the capacity of machines to carry out jobs that are traditionally performed by people.
Let’s analyze each of these ideas in depth so that you can more clearly comprehend how AI and machine learning vary from one another.
What Is Artificial Intelligence?
AI, or Artificial Intelligence, is a rapidly evolving field at the intersection of computer science and cognitive science. It focuses on developing intelligent machines capable of performing tasks that typically require human intelligence, such as learning, problem-solving, speech recognition, and decision-making. AI has revolutionized various industries and continues to shape the future of technology and society.
Machine Learning
Machine learning is a subset of artificial intelligence that uses algorithms to identify patterns and make predictions based on data. It’s a powerful tool that can help organizations automate processes, increase efficiency, and make better decisions. From healthcare to finance, education to transportation, machine learning applications are being used to solve complex problems and improve outcomes.
The differences between AI and Machine Learning
Now that we are familiar with AI and machine learning let’s examine how they differ from one another.
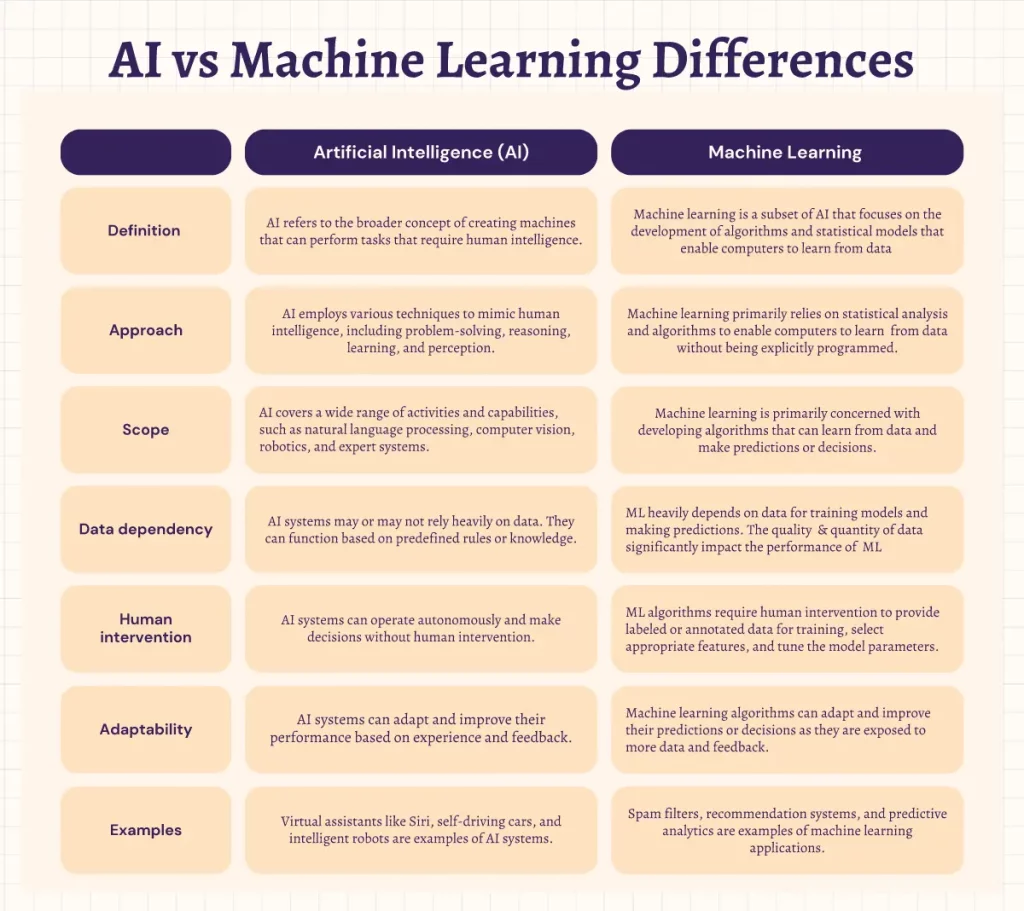
- Nature:
The nature of AI and machine learning is where the primary difference lies. The term “artificial intelligence” (AI) covers various disciplines, including computer vision, natural language processing, and machine learning. Machine learning, on the other hand, is a subset of AI that focuses on computers finding patterns in data and making decisions based on that data.
To carry out specific responsibilities, AI devices follow pre-programmed rules.
On the other hand, machine learning algorithms, on the other hand, make use of statistical approaches to let computers learn from data and enhance their ability at a specific activity through practice.
2. Input
AI robots must be fed with structured data because they are programmed to carry out specified tasks. On the other hand, machine learning algorithms are more versatile and flexible as they can learn from structured and unstructured data.
3. Purpose
AI aims to build machines that can perform tasks that generally need human intelligence. The goal of machine learning, in contrast, is to create algorithms that let computers learn from data and improve their performance on specific tasks.
4.Scope:
AI encompasses various techniques and technologies, including machine learning, natural language processing, computer vision, robotics, and more. It is a broad umbrella term encompassing the development of intelligent systems capable of performing tasks that typically require human intelligence. Machine learning, however, is a subset of AI that deals explicitly with algorithms and models that learn from data to make predictions or take actions. Machine learning focuses on the training aspect of AI, while AI covers a broader spectrum of techniques and applications.
5.Learning Capability:
AI systems can learn from various sources, including data, experience, or explicit programming. This learning capability allows AI systems to adapt and improve their performance over time. Machine learning algorithms, as a specific subset of AI, primarily focus on learning from data. They are designed to automatically improve their performance without explicit programming by detecting patterns and relationships in the training data. Machine learning algorithms are specifically designed to process and learn from data to make accurate predictions or take appropriate actions.
6.Human Involvement:
AI systems can often perform tasks autonomously without requiring human intervention. These systems can execute predefined rules, logical reasoning, or statistical probabilities to make decisions. However, in the case of machine learning, human involvement is necessary during the training phase. This involvement includes providing labelled data, which serves as the training set, and defining the learning objectives. The training process involves humans in curating the data and guiding the algorithm to learn the desired patterns and relationships.
7.Data Dependency:
AI systems can operate with or without large amounts of data, depending on the specific application and technique being employed. Some AI techniques, such as rule-based systems, may not require extensive data to function effectively. In contrast, machine learning heavily relies on data for training models and improving their accuracy. The availability and quality of data greatly influence the performance and predictive capabilities of machine learning models. The more diverse and representative the training data, the better the machine learning model can generalize and make accurate predictions.
8.Flexibility:
AI systems are designed to handle various tasks and adapt to new situations. They are generally more flexible in addressing multiple problem domains. AI techniques, such as natural language processing and computer vision, can be applied to different contexts and scenarios. On the other hand, machine learning algorithms are more specialized and focused on solving specific problems within a defined domain. They are designed to excel at particular tasks, such as image recognition, speech recognition, or fraud detection, but may lack the same level of adaptability as broader AI systems.
9.Decision-Making:
AI systems can decide based on predefined rules, logical reasoning, or statistical probabilities. These systems can follow predefined guidelines to perform tasks or evaluate complex scenarios based on logical rules. Machine learning models, on the other hand, make decisions based on patterns and correlations they have learned from the training data. These models generalize from the provided data to predict or take actions in real-world scenarios. Machine learning algorithms excel at recognizing patterns in data and leveraging those patterns to make informed decisions.
10.Interpretability:
AI systems often lack interpretability, meaning it can be challenging to understand the reasoning behind their decisions. This is particularly true for complex AI models such as deep learning neural networks, whose inner workings are highly complex and abstract. While these models can provide accurate predictions, it may be difficult to explain how and why a particular decision was reached. In contrast, machine learning models, depending on the algorithm used, can offer some level of interpretability. Specific machine learning techniques, such as decision trees or linear regression, provide explanations or feature importance measures that help understand how the model arrived at a specific prediction or decision.
11.Complexity:
AI systems can be highly complex, incorporating multiple components and technologies to achieve their functionality. AI encompasses various techniques, including machine learning, natural language processing, computer vision, and robotics, which may involve intricate algorithms and architectures. The complexity of AI systems arises from the need to simulate human intelligence and perform complex cognitive tasks. Machine learning algorithms, on the other hand, can range from simple to complex. While some algorithms, like linear regression, are relatively straightforward, others, such as deep learning neural networks, can be highly intricate and require significant computational resources.
12.Application Areas:
AI has diverse applications across numerous domains. It powers technologies like self-driving cars, virtual assistants, fraud detection systems, recommendation engines, and more. The broad scope of AI enables its integration into various sectors, including healthcare, finance, manufacturing, and entertainment, to name just a few. Machine learning, within the AI landscape, finds applications in specific areas such as image recognition, natural language processing, predictive analytics, personalized marketing, and anomaly detection. These applications leverage machine learning algorithms to extract insights, make predictions, and automate decision-making processes.
The Applications of Machine Learning
Machine learning has the power to transform industries by providing insights that were previously impossible to obtain. Machine learning algorithms may identify patterns and make predictions with high accuracy by analyzing vast quantities of data.
This has applications in a wide range of fields, including:
- Healthcare
Healthcare organizations are adopting machine learning to lower costs and enhance patient outcomes. It can be used to analyze patient data, identify trends, and predict disease progression.
Machine learning algorithms can also be used to develop personalized treatment plans that take into account a patient’s medical history, genetics, and lifestyle.
One example of machine learning in healthcare is the development of personalized treatment plans for cancer patients. Machine learning algorithms can analyze patient data, including genetic information and medical history, to identify the most effective treatment options for individual patients. This can help improve patient outcomes while also lowering expenditures.
2. Finance
In the finance industry, machine learning applications are being used to detect fraud, predict market trends, and improve risk management.
Machine learning algorithms can analyze large amounts of financial data to identify patterns and predict future market trends. This can help financial organizations in making better investment decisions and reducing risk.
Like, Machine learning is being used in finance to improve fraud detection. Machine learning algorithms can analyze financial data to identify fraud patterns and flag suspicious transactions. This can help financial institutions detect and prevent fraud more quickly and efficiently.
3.Education
In education, machine learning is being used to personalize learning experiences for students. Machine learning algorithms can analyze student data to identify areas of strength and weakness and develop personalized learning plans. This can help pupils learn more effectively and get better academic results.
One example of this is the use of adaptive learning technologies, which use machine learning algorithms to analyze student data and develop personalized learning plans. This can help students learn more effectively and improve academic outcomes.
4. Transportation
Machine learning is being used in transportation to improve safety, reduce congestion, and optimize routes. Machine learning algorithms can analyze traffic patterns and predict traffic flow, allowing transportation planners to develop more efficient ways and reduce traffic jams.
Machine learning is also being used in the development of autonomous vehicles, which have the potential to transform transportation.
One example of this is the use of intelligent transportation systems, which use machine learning algorithms to analyze traffic patterns and predict traffic flow. This can help transportation planners develop more efficient routes and reduce congestion.
5. Retail
To enhance client experiences and boost revenue, machine learning is employed in the retail industry. Machine learning algorithms can analyze customer data to identify buying patterns and develop personalized marketing campaigns. This can help retailers improve customer loyalty and increase sales.
Applications of Artificial Intelligence
- Personal Assistants: AI-powered personal assistants such as Siri, Alexa, and Google Assistant aid users with tasks such as texting, setting reminders, and making phone calls.
- AI can be used in healthcare to analyze patient data, diagnose ailments, and design personalized treatment regimens.
- Robotics: Artificial intelligence (AI) can be utilized to control robots, which can be used in industries such as manufacturing, logistics, and transportation.
- AI in gaming can create intelligent and challenging opponents for gamers.
- Fraud Detection Using Machine Learning Algorithms: Machine learning algorithms can detect fraudulent actions in the banking, insurance, and e-commerce industries.
- Machine learning algorithms can create recommendation systems that propose items, films, or music to people based on their tastes.
- Machine learning algorithms can identify objects, faces, and scenes in photos and videos.
- Natural Language Processing: Machine learning algorithms can be used to analyze and understand human language, allowing chatbots and virtual assistants to communicate with humans.
Conclusion
Finally, artificial intelligence and machine learning are different terms commonly employed interchangeably. Machine learning is a subset of AI, which is a broader concept.
Machine learning refers to machines learning from data, understanding patterns, and making decisions based on that data. In contrast, AI refers to robots’ capacity to perform tasks that would ordinarily need human intelligence.
Understanding the distinction between AI and machine learning is essential for firms seeking to gain a competitive advantage by using these technologies. These technologies, which automate manual procedures, increase productivity, and improve decision-making, can help businesses improve their business outcomes.
FAQ’S
What is the main difference between AI and machine learning?
Machine learning is a subset of AI, which is an additional idea. Machine learning is a subset of AI that involves machines learning from data, identifying patterns, and making judgments based on that data. AI refers to the ability of devices to perform tasks that typically require human intelligence. In contrast, machine learning is a subset of AI that involves machines learning from data, detecting patterns, and making decisions based on that data.
Can AI exist in the absence of machine learning?
Yes, AI can exist in the absence of machine learning. Without learning from data, AI can be designed to execute specific tasks.
What are the main advantages of AI and machine learning?
AI and machine learning can help to automate manual activities, increase productivity and accuracy, improve decision-making, and save expenses.
Are AI and machine learning interchangeable?
No, AI and machine learning are not the same thing. Machine learning is a component of the broader concept of artificial intelligence (AI).
What are some of the most popular uses of AI and machine learning?
Some of the most common applications of machine learning and artificial intelligence are personal assistants, healthcare, robots, fraud detection, recommendation systems, picture acceptance, and natural language processing.
What are the potential future uses of AI and machine learning?
AI and machine learning has enormous future potential. They can seriously affect areas like healthcare, banking, education, and manufacturing.